Sangwoong Yoon
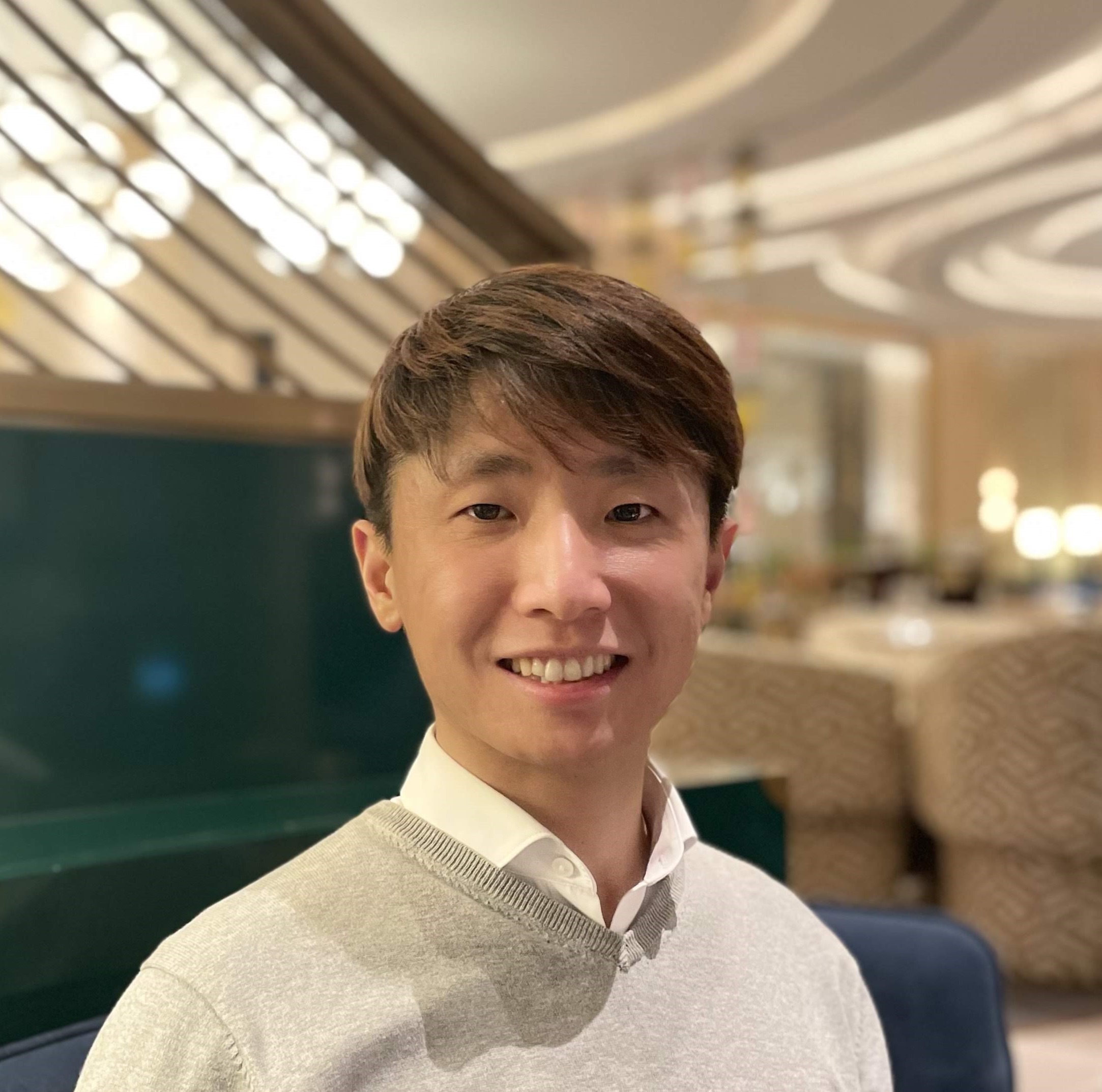
I currently serve as an AI Research Fellow at Korea Institute for Advanced Study (KIAS). Also, I am a visiting scholar in Prof. Ilija Bogunovic's Lab at University College London.
I obtained my PhD in Mechanical Engineering from Robotics Laboratory at Seoul National University, under the supervision of Prof. Frank C. Park. Before PhD, I earned a master's degree in Neuroscience from the same university, guided by Prof. Byoung-Tak Zhang.
Beyond academia, I have accumulated practical industrial experience. I worked at Amazon as an applied scientist intern and Kakao Brain as a research scientist intern. I also held full-time positions as a machine learning scientist at Haezoom Inc. and Saige Research, both are start-up companies based on Seoul.
My academic endeavors have earned me several accolades. I received the Outstanding Doctoral Dissertation Award from Department of Mechanical Engineering at Seoul National University. I also garnered recognition through the Qualcomm AI Fellowship Korea 2021 and Youlchon AI Stars Fellowship 2021. Furthermore, my work, Normalized Autoencoder, was awarded with both Best Poster Award and Most Popular Poster Award at the Machine Learning Summer School 2021 (MLSS 2021).
My research is centered on understanding probabilistic learning principles and building their practical applications. I am deeply engaged with generative models, especially energy-based and diffusion models, exploring their potential in areas such as out-of-distribution detection, reinforcement learning, robotics, and decision making under uncertainty.
I am open for collaboration and also looking for postdoc opportunities outside Korea. Please contact me at sangwoong24yoon at gmail.com. Check out my cirriculum vitae and Google Scholar.
Research Highlights
(1) Exploiting low-dimensional structure of data in energy-based modeling and its application to anomaly / out-of-distribution detection.
- Normalized Autoencoders [link] [code] [web demo].
- Manifold Projection-Diffusion Recovery [link].
(2) Investigating the connection between generative modeling and inverse reinforcement learning.
- Diffusion by Maximum Entropy Inverse Reinforcement Learning [link].
(3) Applying ML on natural sciences, engineering, and robotics (mostly done with external collaborators)
- Tracking hurricane trajectories [link].
- Modeling constitutive equations in complex fluids using RNNs [link].
- Detecting collisions on a robot using an autoencoder [link].
- Analyzing impinging jet flames [link].
- Understanding spatial patterns of fibrosis in liver [link]
News
- (21/07/2024 - 27/07/2024) Attending ICML 2024.
- (02/07/2024) Preprint Maximum Entropy Inverse Reinforcement Learning of Diffusion Models with Energy-Based Models is now public!
- (1/07/2024 - 30/09/2024) Visiting University College London for research collaboration with Ilija Bogunovic.
- (28/06/2024) The result of collaboration with Mayo Clinic is published in Science Advances.
- (23/02/2024) Invited talk at Cambridge Image Analysis Group, University of Cambrdige.
- (27/12/2023 - 28/12/2023) Invited talk at 4th KCMS-Theory Workshop.
- (10/12/2023 - 17/12/2023) Attended NeurIPS 2023.
- (31/10/2023 - 03/11/2023) Co-organized KIAS-HYU Summer School on Diffusion Models.
- (29/10/2023) A paper is accepted at NeurIPS Workshop on Diffusion Models 2023.
- (22/09/2023) Two posters are accepted at NeurIPS 2023.
- (01/09/2023) Invited talk at ExploreCSR program hosted by Google and SNU Graduate School of Data Science.
- (29/08/2023) Recieved Outstanding Doctoral Dissertation Award from Department of Mechanical Engineering, Seoul National University.
- (02/08/2023) Data-driven constitutive model of complex fluids using recurrent neural networks is published in Rheological Acta.
- (04/03/2023 - 19/03/2023) Visited Institute for Theoretical Physics, Heidelberg University.
- (06/06/2022 - 09/09/2022) Internship at Amazon in Seattle.